Industrial Asset Data Integrity + AI -- Top 3 Takeaways
On September 19, 2023, we hosted a webinar — together Adam Weiss from Datamura Solutions — to discuss the challenges with industrial asset data integrity and explore how AI can help.
Conversation Top 3 Takeaways
#1
Industrial asset data integrity deals with the state of accuracy, consistency & reliability of data associated with an organization’s physical assets such as equipment, machinery, infrastructure & facilities. It involves ensuring that the information associated with these assets is correct, up-to-date, trustworthy and can be effectively used for decision-making, compliance, maintenance and other critical operational processes.
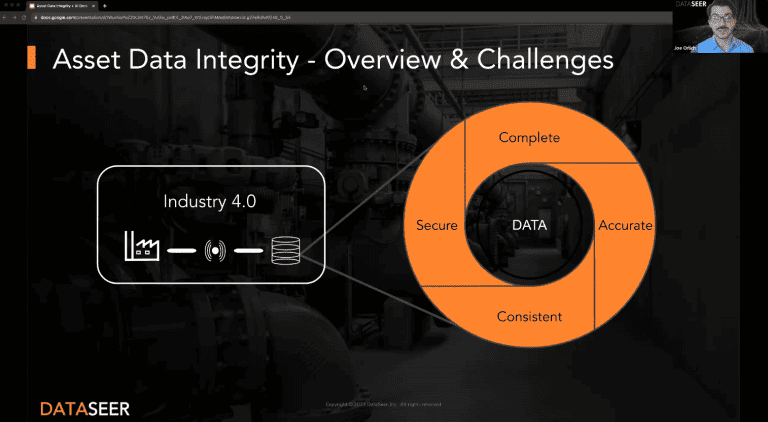
The following areas need to be addressed w.r.t asset data:
- Completeness
- Accuracy
- Security
- Consistency
Areas to consider when working with asset data & maintaining data integrity:
- Data Governance & Architecture
- Data Integration
- Master Data Services
- Data Visualization
- AI & ML
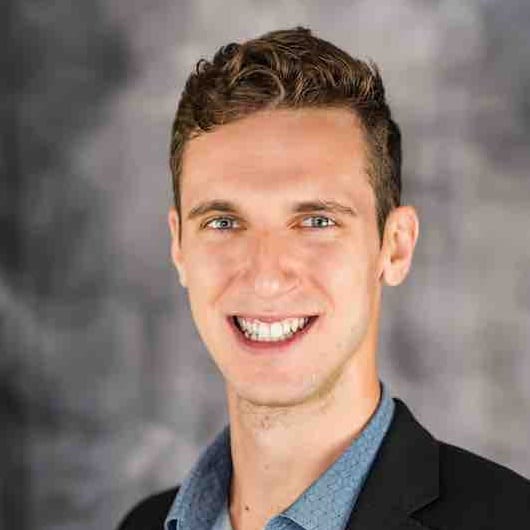
#2
Adam Weiss from Datamura Solutions introduced how decision-making can be powered by intelligent data design and be improved at the macro and micro scales.
Organizations struggle with data deficiency & data apathy. Being data-driven doesn’t mean gathering millions of data samples. This can be unnecessary and distracting to the workforce. This is where thoughtful data design & capture plays a role.
Data selectiveness involves examining the goal at hand, asking yourself with the “Future You” will need, and considering which existing processes need to be changed. Automation can sometimes be leveraged for some processes, e.g. in data collection. By framing the problem in this way, this can help organizations overcome the hesitation to use their own data & benefit from advantages in data-driven decision making.
To help humans “see” and digest data quickly for decision-making, data structures need to be designed to allow for quick & accurate interpretation and understanding. An optimal data capture process will allow an engineer to reduce the mental burden of tedious repetitive “mindless” tasks while also help with complex decision making.
Once collected, data can be used in a variety of ways, e.g. finding correlations & relations, running analytics to support decision making. Types of analytics include:
- Descriptive: What happened?
- Diagnostic: Why did it happen
- Predictive: What will happen
- Prescriptive: What actions should be taken?
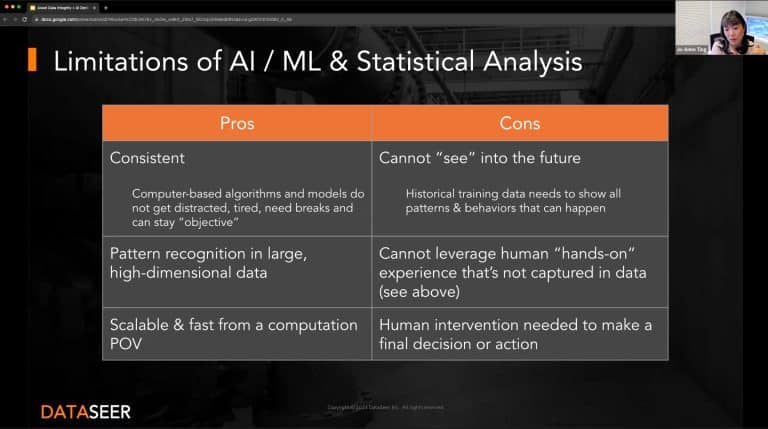
#3
Jo-Anne Ting from DataSeer introduced AI & ML techniques that may come useful depending on the type of industrial data encountered. These range from outlier / anomaly detection and imputation methods in case of IoT sensor streams; to dimensionality reduction techniques in case of high-dimensionality data to focus on relevant clusters of features; and — as part of getting to actions — to text summarization, object classification & time-series forecasting.
Pros & cons of AI / ML + statistical analysis? Computer-based models & algorithms are great since they don’t get tired, distracted and can stay “objective”, but this is also a disadvantage since human domain expertise & experience cannot be leveraged … unless, of course, this is already observed in data or can be captured mathematically.